5 Problems Associated With Machine Learning
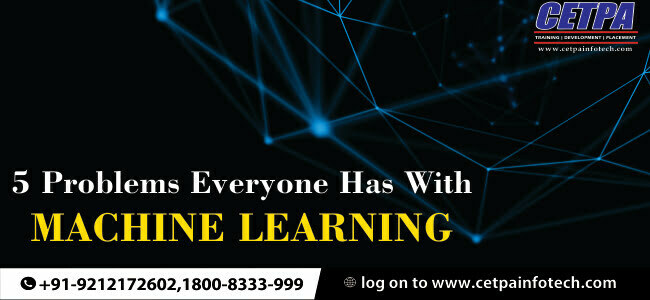
Machine learning offers organizations with the knowledge to make more knowledgeable, data-driven decisions that are quicker and leaner than traditional methods. But, it’s not the mythical or magical process that many build it up to be. Machine learning offers its own set of challenges compared to other methods. In this post, we will discuss 5 problems associated with Machine Learning.
Understanding Which Processes Need Automation
It’s becoming increasingly challenging to isolate facts from fiction in terms of machine learning now. Before you decide on which AI platform to work, you need to decide which problems you’re looking to solve. While machine learning can definitely help automate some processes, not all automation problems require machine learning. To understand, which process to us, join Machine learning Training in Noida.
Beginning without Good Data
The number one problem faced during machine learning starting out with a lack of good data. While enhancing algorithms often consumes most of your time in AI, data quality is essential for the algorithms to function as intended. Dirty data, Noisy data, and incomplete data are the quintessential opponents of ideal machine learning.
Inadequate Infrastructure
Machine learning needs huge amounts of data churning capabilities. Legacy systems usually can’t handle the workload and buckle under pressure. You should check if your infrastructure can manage machine learning. If it can’t, you should look to upgrade, complete with hardware acceleration and flexible storage and machine learning is very popular technology because of these features.
Read More: Is Machine Learning Trendy?
Implementation
Organizations often have analytics engines working with them by the time they choose to update to machine learning. Integrating newer machine learning methodologies into existing methodologies is a difficult task. Maintaining proper interpretation and documentation goes a long way into easing implementation. Machine learning training in Delhi can help you with this.
Lack of Skilled Resources
Machine learning and Deep analytics in their modern form are still new technologies. As such, there is a lack of skilled resources you can hire to manage and develop analytical content for machine learning. Data scientists often need a blend of domain experience as well as in-depth knowledge of science, technology, and mathematics.
These examples should not discourage you from using ML tools to lessen your workloads. The above-given points are some of the common mistakes which marketers should keep in mind when developing ML algorithms and projects. Machine learning models are constantly evolving and the insufficiency can be overcome with exponentially growing real-world data and computation power in the near future. To learn more about this field, join the machine learning course in Noida.
1 Comment
Your blog about the problems in machine learning is so helpful for me……i need more information about this topic so add some more informations about it.